MSc in Actuarial Science and Insurance Analytics (MSc ASI) Sample Class
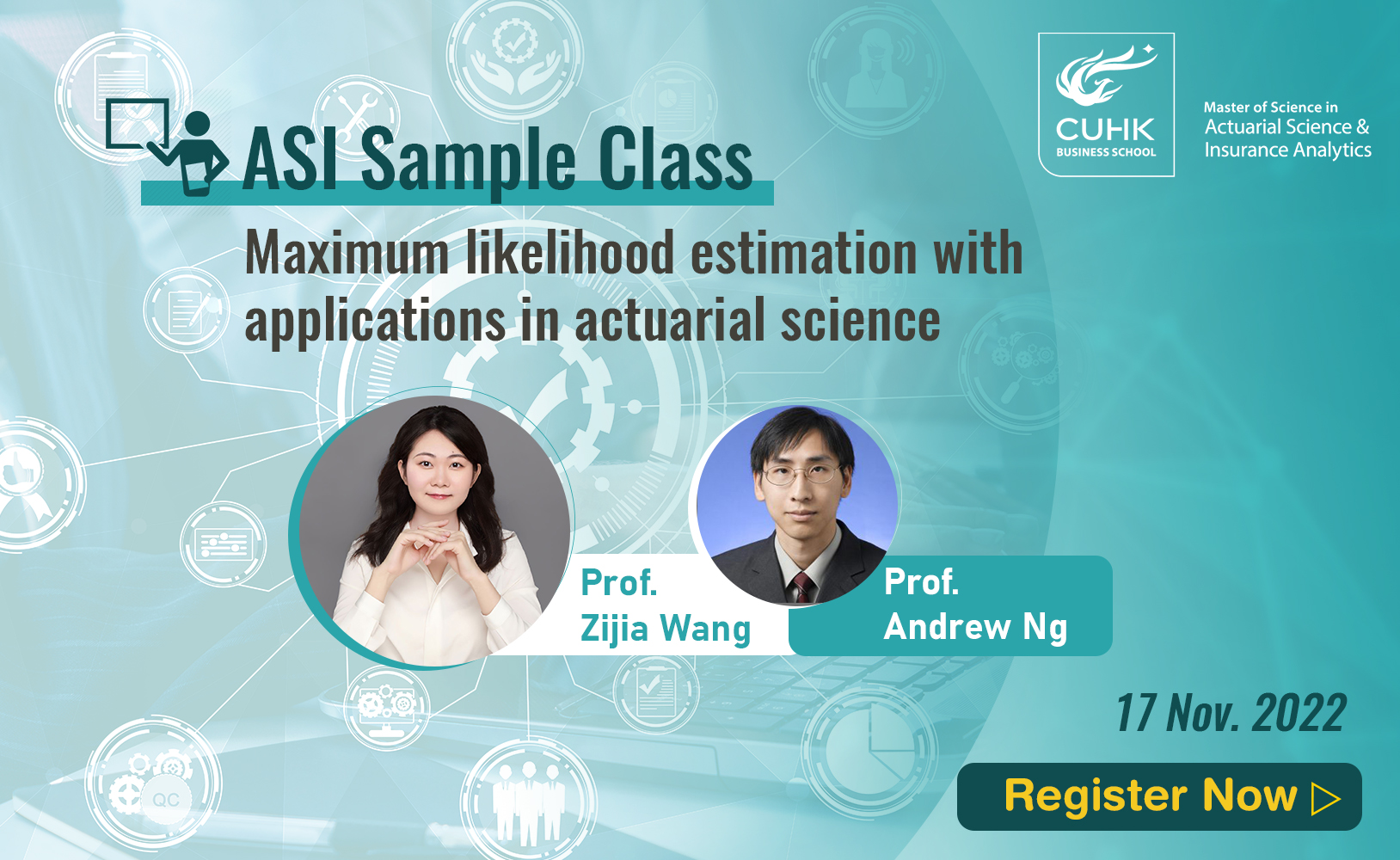
In insurance applications, heavy-tailed distributions such as the Pareto distribution are essential tools for modelling extreme losses. It is particularly important to use as much information as possible to successfully estimate parameters for heavy-tailed distributions. In these cases, estimation by the method of moments and percentile matching tends to perform poorly, mainly because they use only a few features of the data.
In this sample class, we will discuss maximum likelihood estimation (MLE) for losses and frequency models with a few examples. We will consider MLE for complete individual data, complete grouped data, and truncated or censored data under insurance policy adjustments.